
Welcome to the 1st MSDS 2023!
The aims of this symposium are to exchange on the advances and achievements in the field of data sciences, spanning from marketing, financial technology, artificial intelligence, law, education, language, precision medicine and smart governance etc. In particular, the event will bring together speakers from diverse backgrounds to discuss the latest research on data science or data science tools.
1) Data mining and data management;
2) Machine learning algorithms and techniques;
3) Natural language processing;
4) Big data analytics and processing;
5) Data visualization and storytelling;
6) Ethical and legal issues in data science;
7) Applications of data science in various industries.
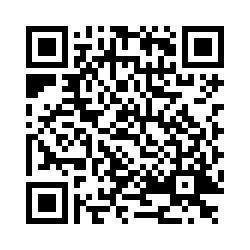
Schedule
From | To | Speaker 講者 |
---|---|---|
09:00 | 09:20 | Opening Ceremony |
09:20 | 09:30 | Group Photo |
09:30 | 10:30 | KEYNOTE Presentation, Prof. Reynold CHENG Data Science for Social Goods: STAR Lab’s Experience |
10:30 | 10:45 | Coffee break |
10:45 | 11:30 | Industrial Talk, Huawei – Mr. YIM Kwok Woon, Jack Leveraging Advanced Intelligent Technology to Power the Next Wave of Digital Transformation |
11:30 | 12:00 | Technical Session 1, Prof. Chao HUANG Robust and Explainable Spatio-Temporal Graph Learning for Smart City |
12:00 | 12:30 | Technical Session 1, Prof. May WANG Revolutionizing Business Innovation: The Power of Creative AI in Data Science |
12:30 | 14:00 | Lunch break |
14:00 | 15:00 | KEYNOTE Presentation, Prof. Jiangliang XU Large-scale Geospatial Analytics: Challenges and Opportunities |
15:00 | 15:30 | Technical Session 2, Prof. Ming Ming CHIU Applying Artificial Intelligence & Statistics to Big Data: Automatic Analysis of Conversations |
15:30 | 16:00 | Technical Session 2, Prof. Minjia TAN Integrative proteogenomic and pharmacological characterization of disease samples identifies therapeutic opportunities |
16:00 | 16:30 | Technical Session 2, Prof. Yun ZHAO Dualism in data protection: balancing the right to personal data and the data property right |
16:30 | 16:45 | Coffee break |
16:45 | 17:15 | Technical Session 3, Prof. Yu LU AI-Driven Research and Practice in Education |
17:15 | 17:45 | Technical Session 3, Prof. Tianji CAI Modelling municipal solid waste generation in Macau: A synthetic approach |
17:45 | 18:15 | Technical Session 3, Prof. Terence POON Opportunities and Pitfalls in Application of High-Throughput Proteomics and Metabolomics Technologies to Disease Marker Discovery |
18:15 | 19:45 | Dinner for invited guest |
Keynotes
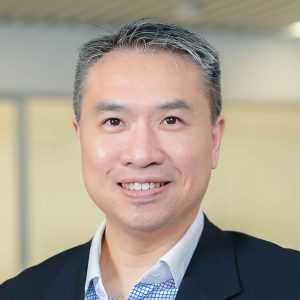
Reynold CHENG 鄭振剛
Professor, The University of Hong Kong
Prof. Cheng received his MSc and PhD degrees from Department of Computer Science of Purdue University in 2003 and 2005. Prof. Cheng is a Professor of the Department of Computer Science in the University of Hong Kong (HKU). His research interests are in data science, big graph analytics and uncertain data management. He was an Assistant Professor in the Department of Computing of the Hong Kong Polytechnic University (HKPU) from 2005 to 2008.
Prof. Cheng received the SIGMOD Research Highlights Reward 2020, HKICT Awards 2021, and HKU Knowledge Exchange Award (Engineering) 2021. He was granted an Outstanding Young Researcher Award 2011-12 by HKU. He received the Universitas 21 Fellowship in 2011, and two Performance Awards from HKPU Computing in 2006 and 2007. He is an academic advisor to the College of Professional and Continuing Education of HKPU. He is a member of IEEE, ACM, ACM SIGMOD, and UPE. He was a PC co-chair of IEEE ICDE 2021, and has been serving on the program committees and review panels for leading database conferences and journals like SIGMOD, VLDB, ICDE, KDD, IJCAI, AAAI, and TODS. He is on the editorial board of IS and DAPD, and was a former editorial board member of TKDE.
Talk Title: Data Science for Social Goods: STAR Lab’s Experience
Talk Abstract
In many metropolitan cities, there is a lack of manpower in social care. In Hong Kong, for example, the elderly care homes report a 70% shortage of employees. To alleviate these issues, recently there is a lot of attention on “data science for social goods”, or the use of technologies for enhancing service quality and streamlining administrative work of social workers. In this talk, I will discuss how the HKU STAR (Social Technology And Research) Lab uses data science technologies to support elderly and family care services. I will first introduce HINCare, a software platform that provides volunteering and cultivating mutual-help culture in the community. HINCare uses the HIN (Heterogeneous Information Network) to recommend helpers to elders or other service recipients, and is now supporting 14 NGOs and 7,000 users. I will also discuss our collaboration with the Hong Kong Jockey Club Charities Trust for developing a novel case management and data analysis system for 40% of the family care centers in Hong Kong. These projects have received an HKICT Award, Asia Smart App Awards, and HKU Faculty Knowledge Exchange Awards.
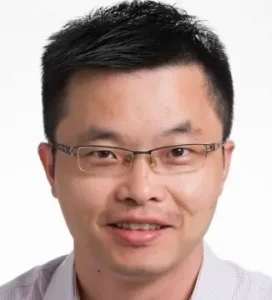
Jianliang XU 徐建良
Chair Professor, Hong Kong Baptist University
Jianliang Xu is the Head and Chair Professor of the Department of Computer Science at Hong Kong Baptist University, where he leads the Database Research Group. His current research interests include big data management, data security & privacy, and blockchain technology. With an h-index of 56, he has published more than 250 technical papers in these areas, most of which appeared in leading journals and conferences such as SIGMOD, VLDB, ICDE, TKDE, and VLDBJ. He is listed among the World’s Top 2% Scientists by Stanford University. He has served as a PC Chair for a number of major international conferences and as an Associate Editor for several esteemed international journals, including IEEE Transactions on Knowledge & Data Engineering and Proceedings of the VLDB Endowment.
Talk Title: Large-scale Geospatial Analytics: Challenges and Opportunities
Talk Abstract
Geospatial analytics has emerged as a crucial tool in diverse fields such as crime science, transportation science, epidemiology, ecology, and urban planning. However, as big geospatial data continues to grow exponentially, conventional geospatial analytics tools encounter efficiency issues when handling large-scale datasets. This poses significant challenges and concerns for domain experts, underscoring the pressing need for more efficient approaches. In this talk, we will delve into the challenges and problems associated with large-scale geospatial analytics. We will share our recent efforts to develop efficient geospatial analytics tools, including kernel density visualization for hotspot detection and K-function for correlation analysis. We will also highlight future opportunities and potential advancements in this field.
Invited Talks
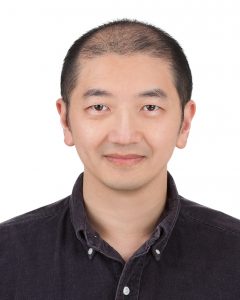
Ming Ming CHIU 趙明明
Chair Professor, The Educational University of Hong Kong
Professor Chiu obtained his Bachelor degree in Computer Science at Columbia University, his master’s degree in education at the Harvard University and his doctorate at the University of California, Berkeley.
Professor Chiu is an analytics expert, integrating statistics, computer science and mathematics to develop theories and test them in diverse fields, including education, psychology, sociology, linguistics, criminology, economics, and management.
Talk Title: Applying Artificial Intelligence & Statistics to Big Data: Automatic Analysis of Conversations
Talk Abstract
As people solve problems together that they cannot do alone, automatic analysis of conversations can inform and enhance their design to aid learning and teaching. Such analyses must traverse the obstacle course of voice transcription, complex categorization, and statistical analysis. Automated transcription feeds automatic categorization via computational linguistics to create a database (Big Data). Automated statistical analysis integrates statistical discourse analysis (SDA) and artificial intelligence. SDA models (a) pivotal actions that radically change subsequent processes and (b) explanatory variables at multiple levels (sequences of turns/messages, time periods, individuals, groups, organizations, etc.) on multiple target actions. The artificial intelligence expert system translates my theory into a statistical model, tests it on the data, interprets the results, (if needed, rewrites itself to execute revised analyses), and prints a table of results. I showcase automated SDA on 321,867 words in 1,330 messages by 17 student-teachers in 13 weekly online discussions of lesson designs.
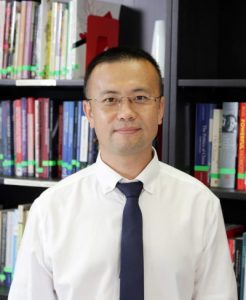
Tianji CAI 蔡天驥
Professor, The University of Macau
Professor Cai received his PhD and Master degree at University of North Carolina at Chapel Hill in 2010.
His research interests concentrate on new forms of data and new methods of analysis. Reflecting on his broad intellectual pursuits, his research topics are diverse, ranging from methodological, such as quantitative methods and data mining, to substantive ones, such as gene-environmental interplay and adolescent health behaviors.
Talk Title: Modelling municipal solid waste generation in Macau: A synthetic approach
Talk Abstract
With the development of urbanization during the past few decades, Municipal Solid Wastes (MSW) generated in urban areas have posed serious challenges to the local environment in many cities around the world, such as Macau. Residential and touristic activities are the two primary sources of solid waste in Macau; however, it is still unclear how and which indicators of these two could be used to predict solid waste generation.
Taking advantage of the data published by government agencies in Macau from 2010 to 2021, our study explored the possibility of using publicly available data to predict the trend of monthly MSW generation in Macau. In particular, we adopted a machine learning strategy and compared six predictive models that implement both conventional and state-of-art machine learning methods.
Our results indicate that the Generalized Additive Model outperformed the rest of the models in predicting MSW generation in Macau and is more suitable when data present strong seasonality and potential domain shift. Consistent with previous literature, both population-level and household-level factors contributed to MSW generation, including population density, income level, household energy consumption, and household size. In addition, our results support that tourism activities — especially tourists’ spending on non-traded goods and services —positively affected MSW generation
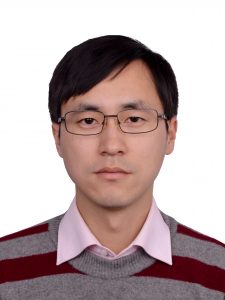
Minjia TAN 譚敏佳
Professor, Shanghai Institute of Materia Medica Chinese Academy of Sciences
Talk Title: Integrative proteogenomic and pharmacological characterization of disease samples identifies therapeutic opportunities
Talk Abstract
Proteins and post-translational modifications (PTMs) play
fundamental roles in disease development. Yet current understanding of the cancer
proteome and therapeutic approaches is by far limited. We conducted a
comprehensive whole exome and transcriptome sequencing, proteomic and
phosphoproteomic analyses of several types of clinical tumor samples and cancer
cell lines, including lung adenocarcinoma, KRAS mutant cancer and acute myeloid
leukaemia. Integrative multi-omics analysis identified the molecular
heterogeneity of these cancers, that is closely related with clinical
characteristics. A large-scale ex vivo drug response profiling, together with
the integration of publicly available drug sensitivity information, identified a
panel of drug combinations which showed therapeutic potentials against certain
subsets of these cancers.
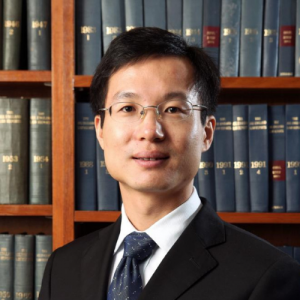
Yun ZHAO 趙雲
Professor, University of Hong Kong
Dr. Zhao obtained his PhD from Erasmus University Rotterdam, an LLM from Leiden University and an LLM & LLB from China University of Political Science and Law.
Dr. Zhao is currently Standing Council Member of Chinese Society of International Law; Council Member of Chinese Law Society; Council Member of Zhuhai International Court of Arbitration; and Chair Professor at Xiamen University (2020-2023). He is listed as an arbitrator in several international arbitration commissions. He sits in the editorial teams of several academic journals and has published widely on various topics including, in particular, Dispute Resolution and Space Law.
Talk Title: Dualism in data protection: balancing the right to personal data and the data property right
Talk Abstract
The presentation explores the issues of the right to personal data and data property rights in the context of commercial transactions involving big data. The analysis herein attempts to break through the traditional concept of ‘property’ with the aim of helping to develop a modern information society in China, devise a property law theory suitable for the big data era, and improve the level of protection afforded rights and legitimate interests in data. To date, no comprehensive study has focused on developing a proper understanding of the concept of ‘data property rights’, and hence we lack the solid theoretical support needed for the construction of a proper protective system for such rights. The presentation examines the rules pertaining to data property rights, thereby enriching the theory of data property rights. It also offers a thorough discussion of how to construct a data property protection system.
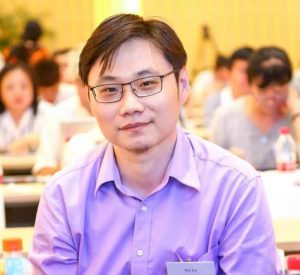
Yu LU 盧宇
Associate Professor, Beijing Normal University
Professor LU received the Ph.D. degree from National University of Singapore in computer engineering, and B.S. degree from Beijing University of Aeronautics and Astronautics (Beihang University).
His current research interests sit at the intersection of artificial intelligence and educational technology, including learner modeling, educational robotics, intelligent tutoring system and educational data mining.
Talk Title: AI-Driven Research and Practice in Education
Talk Abstract
Artificial intelligence (AI) is the technology that enables machine to think or act in a more “human” way to perform tasks that normally require human intelligence. Driven by the fast advancement of AI technologies (e.g., machine learning and generative AI), researchers and practitioners in education , utilizes AI as a powerful tool to support teaching and learning. In this talk, I will talk about how AI influences education from multiple perspectives, including the ongoing AI-driven research and practice for the large amount of students and teachers. I will also discuss the key research issues and promising future of AI in education (AIED) domain.
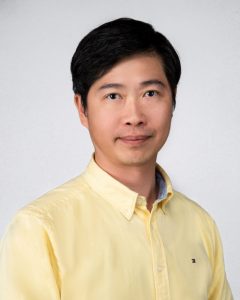
Terence POON 潘全威
Associate professor, The University of Macau
Bio: Dr. Terence C.W. Poon is an Associate Professor at the University of Macau. He obtained his PhD degree in Pathological Sciences from the Chinese University of Hong Kong and his M.Sc. degree in Bioinformatics from the University of Manchester, UK.
His research focuses on clinical proteomics, mass spectrometry, and disease marker discovery. He published 82 articles in SCI journals, such as Gastroenterology, Clinical Chemistry and Clinical and Translational Medicine. Currently, he is the President of Asia Oceania Human Proteome Organization, and he is the Vice-President of China Human Proteome Organization. He is the associate editor of the journal, Clinical Proteomics.
Talk Title: Opportunities and Pitfalls in Application of High-Throughput Proteomics and Metabolomics Technologies to Disease Marker Discovery
Talk Abstract
Discovery of reliable clinical biomarkers for classifying patients into subgroups for receiving different effective treatments is crucial in precision medicine. Since year 2000, various proteomics and metabolomics approaches have been applied to discovery of biomarkers for risk assessment, prediction, screening, diagnosis, monitoring, or prognosis of human diseases as well as for predicting or monitoring response to therapy. Despite more than 20 years of global research efforts, biomarkers from only one proteomics study have been successfully translated into an FDA-approved test in United States. In certain extent, this reflects the presence of difficulties in finding “genuinely useful biomarkers for clinical practice”. Collection of biological specimens from study groups is the first step in biomarker studies. The composition of the study groups and the specimen collection time points depend on the study design. Single-center case-control design and single-center cohort design are the most used study designs for biomarker discovery. However, these two simple study designs could easily result in false biomarkers that are only associated with hidden/known confounding factors. With a proper study design, this pitfall can be avoided, leading to the discovery of reliable biomarkers.
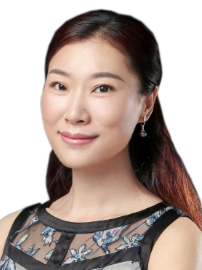
May WANG 王莹
Associate professor, Beijing Normal University – Hong Kong Baptist University United International College
Professor Wang received her Ph.D. in the School of Business from The University of Hong Kong and her M.Phil. in System Engineering and Engineering Management from The Chinese University of Hong Kong.
Her research interests include Big data in E-Commerce, FinTech, recommendation agents, human-computer interaction. Her works have been published in Information Systems Research and Management Science, among others.
Talk Title: Revolutionizing Business Innovation: The Power of Creative AI in Data Science
Talk Abstract
Generative AI (GenAI) has been transforming the landscape of business. This talk explores the transformative potential of generative artificial intelligence in revolutionizing business strategies and practices. With specific examples in marketing applications, GenAI drives greater effectiveness in the customer experience through hyper-personalization, growth through the right analytics and automation, and productivity. The talk presents a comprehensive review and analysis of the evolution of marketing technologies in the context of AI advancements, revealing a three-stage trajectory from a human-centric pre-AI era, through a data-driven post-AI era, to the dynamic and creative post-GPT era. It further identifies critical gaps in current marketing practices and research, particularly around GenAI’s impacts across various business strategies and behaviors. It highlights the need for mindful adoption of GenAI in business practice and the development of business research on GenAI. Generative AI tools present promising opportunities for enhancing consumer engagement, personalizing shopping experiences, automating business analytics, and dynamic content creation, their utilization must be ethical, informed, and considerate of demographic-specific implications. This talk provides valuable insights for future investigations aimed at understanding GenAI’s role in the future of business research, while guiding practitioners towards effective and responsible GenAI integration in their business strategies.
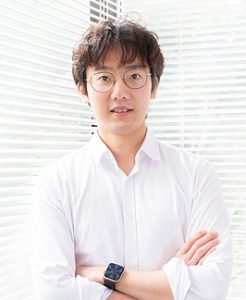
Chao HUANG 黃超
Assistant Professor, University of Hong Kong
Professor Huang received his Ph.D. in Computer Science from the University of Notre Dame in USA. He is an Assistant Professor at the Department of Computer Science at the University of Hong Kong (HKU).
His research focuses on developing novel machine learning frameworks to tackle various challenges in Data Mining, Information Retrieval, Spatial-Temporal Data Analytics, User Behavior Modeling, Recommendation, Graph Mining, and Deep Representation Learning.
Talk Title: Robust and Explainable Spatio-Temporal Graph Learning for Smart City
Talk Abstract
Advancements in remote sensing technologies and large-scale computing infrastructure have led to an unprecedented volume of spatio-temporal data in various fields, including transportation, environmental science, and public security. Effectively harnessing this diverse range of spatio-temporal data calls for the integration of human-centered machine learning techniques with the rich spatio-temporal information available. However, the efficacy of data-driven methods is heavily dependent on the quality of labeled training data, which cannot always be guaranteed in the era of big spatial-temporal data. In addition, in urban computing applications, it is insufficient to merely develop accurate predictive models. Providing human-readable explanations for predictions is equally vital, especially in interdisciplinary domains. To address these challenges, this presentation aims to introduce research that advances the analysis of large-scale spatio-temporal data by developing robust, interpretable, and expressive spatial-temporal graph learning frameworks. This presentation aims to introduce research that advances the analysis of large-scale spatio-temporal data towards robust, interpretable, and expressive spatio-temporal graph learning frameworks.
Industrial Talks
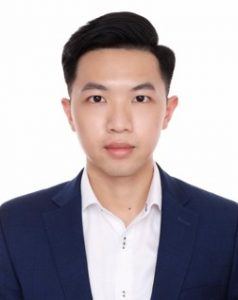
Jack YIM
Cloud Solutions Architect, Huawei
He have extensive experience in the field of Computer & Data Engineering, specifically in the Cloud & AI industry. His expertise spans from research and development to the practical application of AI in various aspects, with a particular focus on Computer Vision (CV) and Natural Language Processing (NLP). Additionally, he have been actively involved in Cloud Computing, specifically in the area of Application Modernization.
Talk Title: Leveraging Advanced Intelligent Technology to Power the Next Wave of Digital Transformation
Talk Abstract
Jack Yim is now a Cloud Solutions Architect of HUAWEI, experienced in the research and development of Artificial Intelligence, Data analysis and Cloud Computing. He will share trend and modern technology for intelligent technology about AI and Big Data
Committee
Chair
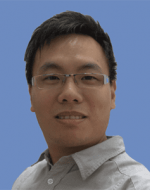
Prof. Leong Hou U, FST
余亮豪 教授
Members
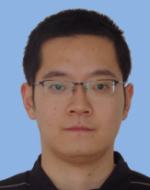
Prof. Dingqi YANG, FST
楊丁奇 教授
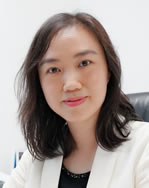
Prof. Cheris Wing Chi CHOW, FBA
周詠芝 教授
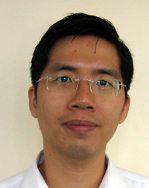
Prof. Henry Chun Kwok LEI, FBA
李振國 教授
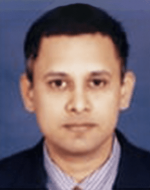
Prof. Muruga Perumal RAMASWAMY, FLL
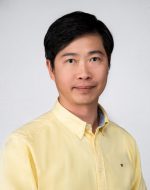
Prof. Chun Wai POON, FHS
潘全威 教授
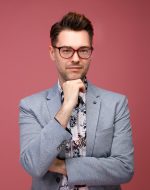
Prof. Barry Lee REYNOLDS, FED
雷貝利教授
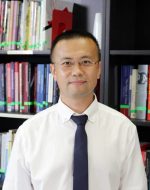
Prof. Tianji CAI, FSS
蔡天驥 教授
Venue
Date: 04 September, 2023
Address: Ground floor Function Hall, N1 Building – University of Macau
Map: UM-Map
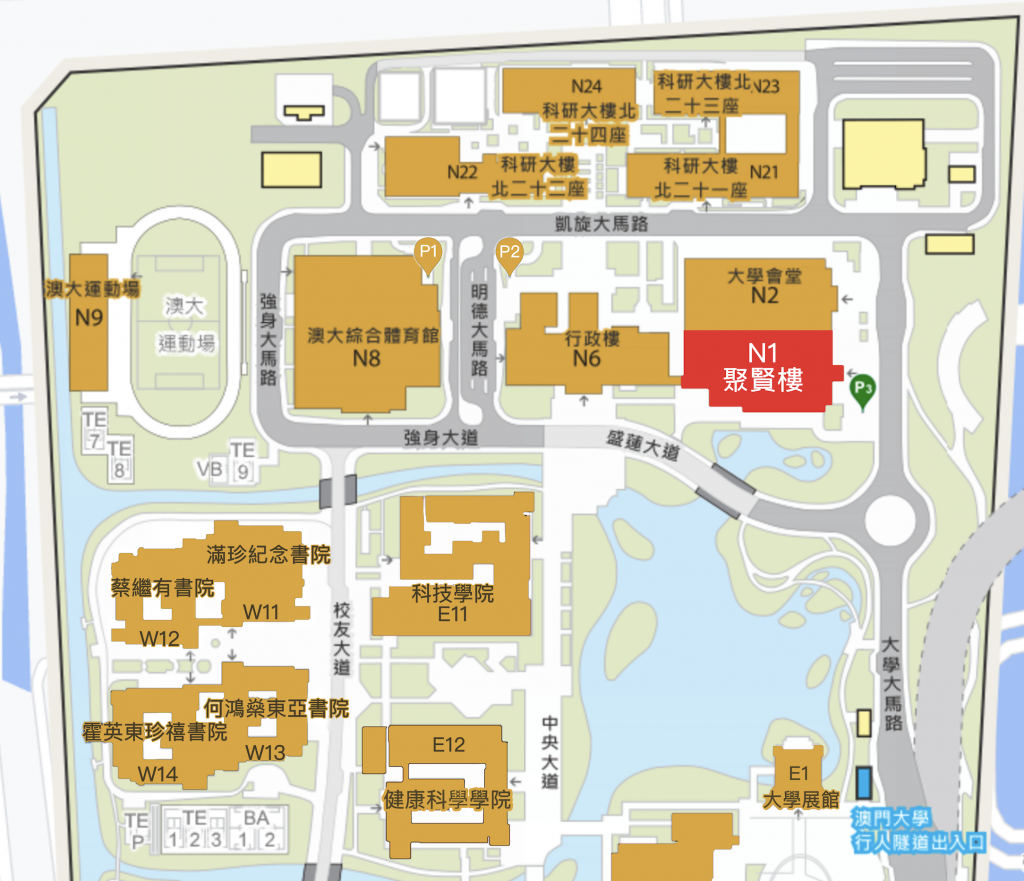